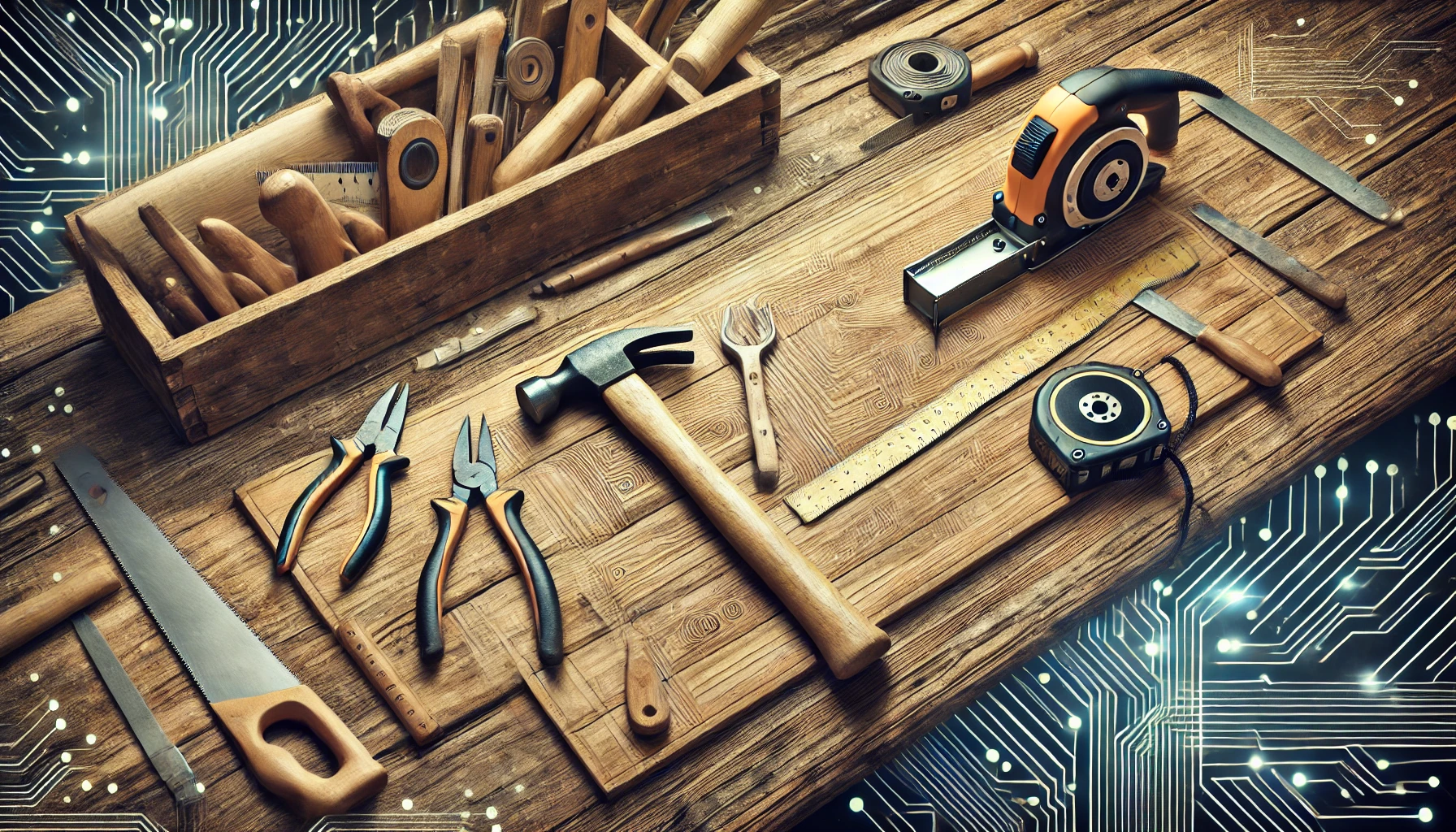
Do we even need ML?
Having worked in the industry for 6 years now, I’ve seen machine learning projects succeed but, most importantly, I’ve seen many more fail - I even failed some of them myself due to inexperience and/or poor judgement. Although each failure has its own story, reasons and learnings, some common denominators always exist. One such important denominator can be “boiled down” to a single assertion that - as an ML engineer - I am particularly fond of:...